Your basket is currently empty!
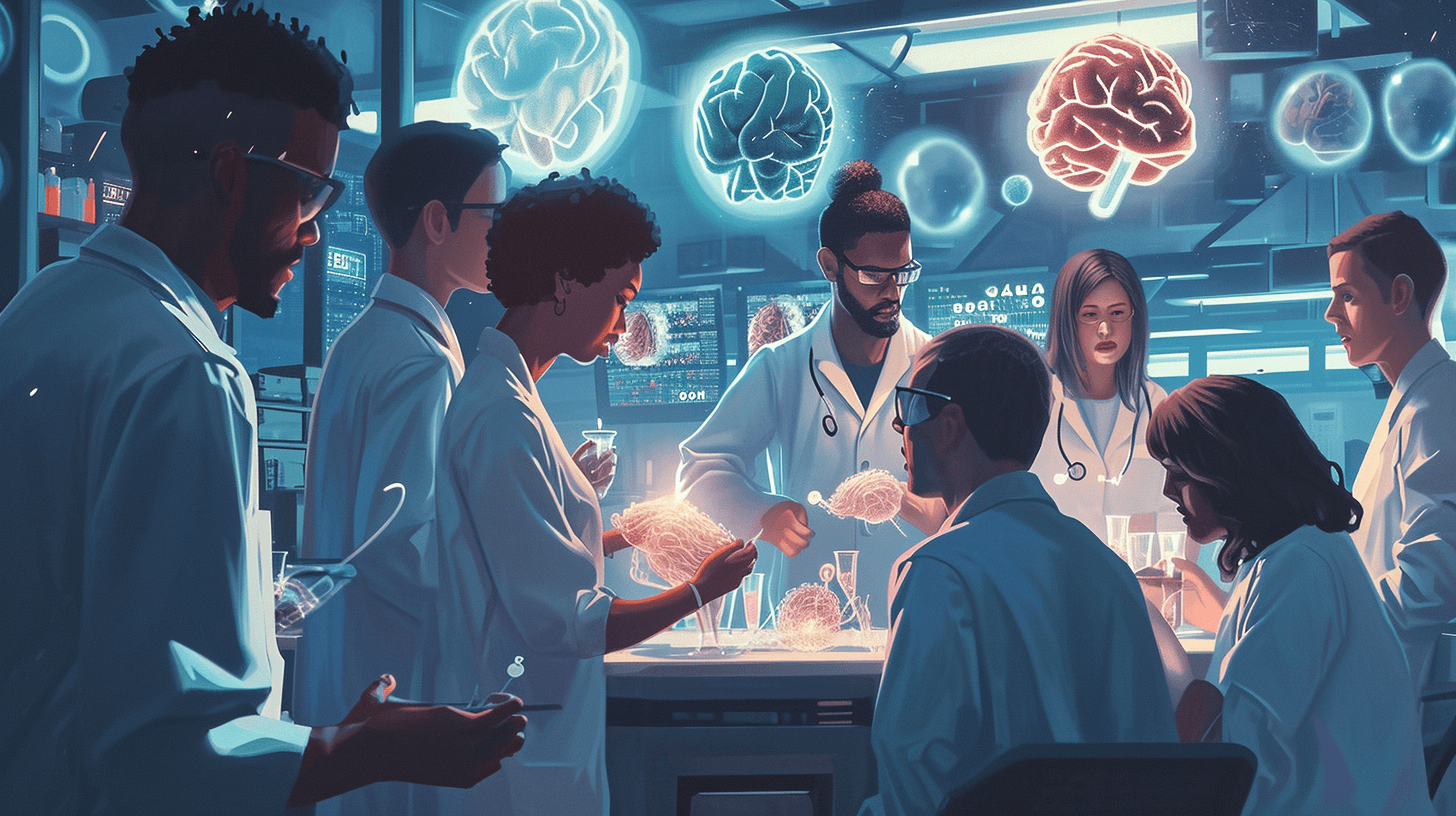
In the world of brain diseases, Alzheimer’s is a complex one.
It’s tricky to diagnose and treat because it has so many different symptoms.
However, a new AI model might make this easier.
This cutting-edge technology predicts how Alzheimer’s disease might develop.
It uses deep learning – a type of artificial intelligence – to estimate when someone might show signs of the disease and how it might develop.
It does this by looking at lots of clinical data and brain scans.
This can help to map out the different ways that Alzheimer’s might progress.
For example, it could show up in memory problems first, then difficulty with daily tasks, and finally, changes in mood or behaviour.
This could help doctors spot the signs of the disease earlier and plan their treatment more effectively.
This technology has the potential to transform the way we deal with Alzheimer’s.
It could make clinical trials more accurate, help doctors make better decisions, and improve the care that patients receive.
In the past, I’ve seen similar technologies make a real difference.
For example, AI has been used with great success to predict the development of other diseases like cancer and diabetes.
So, based on this experience, I’d advise doctors and health organisations to embrace this new technology.
It could help them spot the signs of Alzheimer’s earlier and offer more effective treatment.
As the World Health Organisation reports, around 50 million people worldwide have dementia, with nearly 60% living in low- and middle-income countries.
As there are nearly 10 million new cases, this could be a real game-changer in managing and treating Alzheimer’s disease.
Key Takeaways
- Alzheimer’s disease is a neurodegenerative condition with varied clinical presentation.
- Prompt treatment can improve the overall standard of care for AD patients.
- The development of tools to facilitate early diagnosis is necessary.
- The study findings highlighted a model to predict AD progression using real-world data.
Understanding Alzheimer’s Disease Progression
Frequently, understanding the progression of Alzheimer’s disease is crucial in order to develop effective tools for early diagnosis and treatment.
Accurately predicting the progression of the disease can have a significant impact on treatment decisions and patient outcomes.
In a breakthrough study titled ‘Breakthrough AI Model Predicts Alzheimer’s Disease Progression,’ researchers developed a deep learning-based model that assessed the risk of Alzheimer’s disease progression.
The model utilised clinical and MRI data to estimate time-to-conversion and survival clustering of distinct subgroups.
The results showed that the model had a high prediction accuracy, with significant concordance index values.
This model not only improved our understanding of Alzheimer’s disease progression but also has implications for clinical trial design and decision-making.
By identifying clinical and biological patterns, this model provides a realistic representation of individual patient trajectories, allowing for personalised treatment approaches.
Development of the AI Model
The development of the AI model involved a comprehensive analysis of clinical and MRI data to predict the progression of Alzheimer’s disease.
Researchers utilised neuroimaging techniques and training data from the Alzheimer’s Disease Neuroimaging Initiative (ADNI) and Australian Imaging, Biomarkers and Lifestyle (AIBL) cohorts.
The dataset consisted of 587 individuals in the cognitively normal (CN) to mild cognitive impairment (MCI) group, with 119 individuals progressing to MCI and 783 individuals in the MCI to Alzheimer’s disease (AD) group, with 44% developing AD dementia.
Clinical and neuroimaging parameters, such as cortical thickness and grey matter volume, were retrieved and analysed.
The AI model identified patterns with diverse biomarkers and varying advancement rates, demonstrating significant prediction capacity.
This development is a significant breakthrough and has the potential to aid in clinical trial design and decision-making for Alzheimer’s disease.
Evaluation of the AI Model’s Performance
To assess the effectiveness of the AI model, a thorough evaluation was conducted to determine its predictive accuracy and reliability in predicting the progression of Alzheimer’s disease.
Evaluation metrics were used to measure the performance of the model, including hazard ratios (HR) and concordance index.
The results showed that the model had significant prediction capacity and performed well in estimating conversion time in an external validation cohort.
This indicates that the model is able to accurately predict the progression of Alzheimer’s disease based on real-world data.
Additionally, the model identified patterns with diverse biomarkers and varying advancement rates, further enhancing its reliability.
The findings of this evaluation highlight the potential of the AI model to assist in clinical trial design and decision-making, providing a valuable tool for understanding and managing the progression of Alzheimer’s disease.
Insights Into Clinical and Biological Patterns
The breakthrough AI model revealed several significant clinical and biological patterns in predicting the progression of Alzheimer’s disease.
The model identified diverse biomarkers and varying advancement rates, shedding light on the complex nature of the disease.
It was observed that positive amyloid beta or phosphorylated tau status increased the likelihood of developing Mild Cognitive Impairment (MCI) or Alzheimer’s disease (AD).
Additionally, a causal relationship was found between MCI and AD patterns and time-to-disease conversion.
These insights into clinical and biological patterns have important implications for potential therapeutic interventions and future research directions.
By understanding the specific patterns associated with disease progression, clinicians and researchers can identify targeted treatment strategies and design clinical trials that focus on delaying or preventing the onset of MCI and AD.
Further research in this area can lead to improved care and outcomes for individuals with Alzheimer’s disease.
Implications of Clinical Practice and Research
The breakthrough AI model’s predictions for Alzheimer’s disease progression have significant implications for enhancing clinical practice and advancing research.
The model’s ability to accurately estimate time-to-conversion and survival clustering of distinct subgroups can greatly inform clinical decision-making.
By identifying diverse biomarkers and varying advancement rates, the model improves our understanding of AD progression and provides a realistic representation of individual patient trajectories.
These predictions can play a crucial role in designing clinical trials, allowing for more targeted recruitment and personalised treatment approaches.
Additionally, the model’s ability to identify causal associations between patterns and AD development can guide future research efforts in exploring underlying mechanisms and potential therapeutic interventions.
Conclusion
In conclusion, the breakthrough AI model presented in this article offers a promising tool for assessing the risk of the progression of Alzheimer’s disease.
By utilising deep learning techniques and analysing clinical and MRI data, the model accurately predicts disease trajectories and provides valuable insights into the clinical and biological patterns associated with Alzheimer’s.
These findings have significant implications for clinical practice and research, particularly in terms of improving patient care and informing decision-making in clinical trial design.